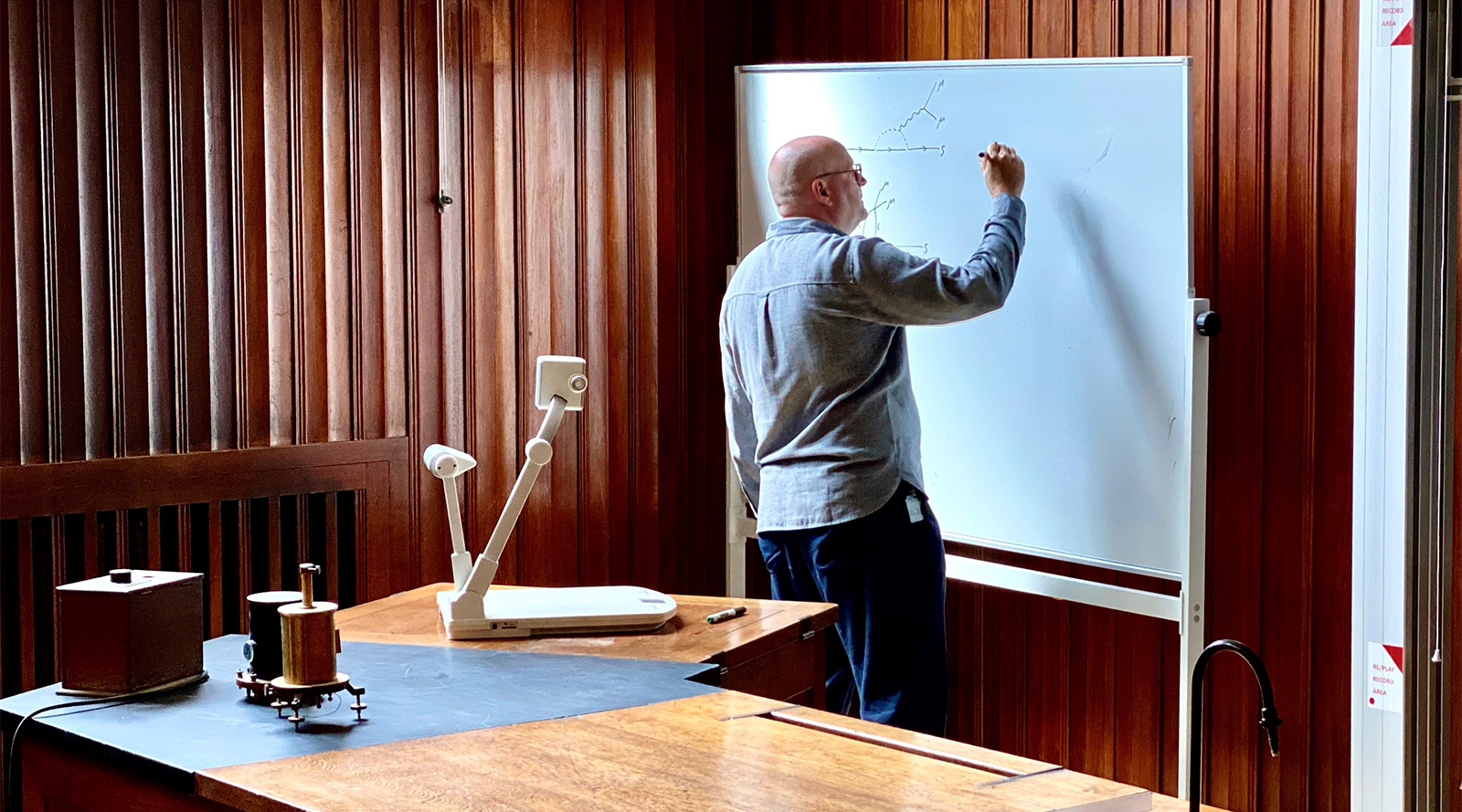
Universities + Research
Create new breakthroughs in machine intelligence to enhance human potential with IPUs.
Create new breakthroughs in machine intelligence to enhance human potential with IPUs.
Material scientists, physicists, roboticists, genomics specialists, epidemiologists, cosmologists, computer scientists and scientific researchers of all kinds are taking advantage of the massively parallel computing power of the IPU to unlock completely new, unlimited directions of research.
The Robot Vision Group at Imperial College London has taken advantage of the IPUs unique architecture to solve classical computer vision problems in a new way using Gaussian Belief Propagation.
“Having led one of the first academic teams to conduct and publish research based on the Graphcore IPU, this is a technology that brings both quantitative and qualitative benefits. We saw the IPU outperforming legacy chip architectures in our computer vision work, but also expanding our understanding of what was computationally possible in this field.”
Andrew Davison, Professor of Robot Vision at Imperial College London
Cosmology researchers at Université de Paris have achieved 4x faster time to train specific neural networks for cosmology applications with IPUs.
A researcher in the Astroparticle and Cosmology Laboratory looked at two deep learning use cases: galaxy image generation from a trained VAE latent space and galaxy shape estimation using a deterministic deep neural network and Bayesian neural network (BNN).
“Many of the data simulations that we have today are based on quite simple galaxy models. And with neural networks, what you can do is also learn more complex shapes of galaxies. And so that’s also very interesting to generate more realistic galaxy images. If the user wants to generate data on-the-fly to train neural networks, I would recommend using IPUs.”
Bastien Arcelin, Researcher at the Université de Paris.
Physicists at the University of Bristol and CERN are tackling challenges in Particle Physics in a new way with IPUs, using Generative Adversarial Networks (GANs) to achieve over 5x performance increase.
“Our work examined the applicability of Graphcore’s IPU to several computational problems found in particle physics and critical to our research on the LHCb experiment at CERN. The capabilities and performance gains that we demonstrated showed the versatility of the IPU’s unique architecture. Moreover, the support that we received from Graphcore has been critical, and remains so, in our ongoing programme of exploring the power of IPUs for processing particle physics’ vast and rapidly increasing datasets.”
Jonas Rademacker, Professor of Physics at the University of Bristol.
UC Berkeley & Google Brain used IPUs for parallel training of deep neural networks with local updates. They found that local parallelism is particularly effective in the high-compute regime.
“The work we did with Graphcore on parallel training of deep networks with local updates illustrates how the IPU’s radically different processor architecture can help enable new approaches to distributed computation and the training of ever-larger models. It is indicative of how Graphcore’s technology does not just deliver quantitatively better performance, against measures such as throughput and latency. The technology is also opening up fundamentally new approaches to the computational challenges that could otherwise hinder the progress of AI.”
Professor Pieter Abbeel, UC Berkeley
University of Massachusetts accelerated Covid-19 modelling using Approximate Bayesian Computation to achieve a 30x speedup on IPUs compared with CPUs and a significant 7.5x speedup compared with GPUs.
Researchers at the University of Oxford are using IPUs to propel us further and faster into the future of quantum computing.
“Creating and maintaining solid state qubits for use in quantum computers is, unsurprisingly, complex. Tuning them and keeping them stable requires analysing and controlling many sensitive variables in real-time. It is a perfect machine learning problem. Our work with Graphcore’s IPU has resulted in dramatic performance gains, thanks to its raw computing power, and the way it manages classic AI challenges such as sparsity. We’re tremendously excited by Graphcore’s next generation IPU technology, and the associated computational power that will propel us further and faster into the future of quantum computing.”
Professor Andrew Briggs, Materials Science, University of Oxford
With 22.6 petaFLOPS of AI compute for both training and inference workloads, the Bow Pod64 is designed for AI at scale.
Learn moreWhen you're ready to grow your processing capacity to supercomputing scale, choose Bow Pod 256 for production deployment in your enterprise datacenter, private or public cloud.
Learn moreA secure IPU cloud service to add state of the art AI compute on demand - no on-premise infrastructure deployment required.
Learn moreSign up below to get the latest news and updates: