Graphcore and our partner Aleph Alpha are unveiling a significant advance in AI compute efficiency, with the sparsification of a 13bn parameter model down to just 2.6bn parameters.
The advanced technique, which removes around 80% of the model’s weights while retaining most of its capabilities, utilizes the IPU’s support for point sparse matrix multiplications - a characteristic of its made-for-AI architecture.
The two companies demonstrated a sparsified variant of Aleph Alpha’s commercial Luminous Chatbot, at SC22 in Texas.
Luminous Base Sparse uses just 20% of the processing FLOPs and 44% of the memory of its dense equivalent. Crucially, its 2.6bn parameters can be held entirely in the ultra-high-speed on-chip memory of an IPU-POD16 Classic, maximising performance.
Parameter pruning
Most AI applications currently make use of dense models, where equal representation and computation is given to all parameters, whether they are contributing to model behaviour or not. As a result, valuable processing time and memory is given over to storing and performing calculations on parameters that have no impact.
Aleph Alpha and Graphcore were able to ‘prune’ the 80% of less relevant weights and re-train the Luminous model using only the important weights – these were represented using the Compressed Sparse Row (CSR) format.
Required compute FLOPS for inference fell to 20% of the dense model while memory use was reduced to 44% as some extra capacity is needed to store both location and value information for the remaining non-zero parameters.
The sparsified model also has a 38% lower energy requirement than its dense counterpart.
Essential efficiency
Sparsification is regarded as an important counterweight to the exponential growth in AI model size and corresponding increase in demand for compute.
Many of the advances in model capabilities for language, vision and multimodal models have been driven by scale. However, training compute increases with approximately the square of parameter count – increasing cost and putting into question the sustainability of operating and scaling ever bigger AI solutions.
For the next generation of models with capabilities even further in the tails of the distribution, sparsification will become crucial, enabling highly specialized sub-models to master dedicated parts of knowledge efficiently.
The use of techniques such as coarse-grained sparsity or selectivity offers the potential to achieve continued rapid advances, with sustainable linear growth in compute.
Such significant downward pressure on the compute needs of AI also expands the commercial potential of artificial intelligence startups such as Aleph Alpha, who are able to deploy highly capable models with minimal compute requirements for customers.
Aleph Alpha
Graphcore and Aleph Alpha first announced their intention to work together on research and model deployment earlier in 2022.
Aleph Alpha is developing original, European-made, multi-modal models including the acclaimed Luminous, which is capable of handling multi-lingual language tasks as well as understanding and answering questions about contexts with any combination of language and visual information.
The original Luminous model is available commercially via API to companies and organisations looking to build AI-driven products and services.
Graphcore and Aleph Alpha are offering commercial applications of their sparsified Luminous Base and 30bn parameter Luminous Extended models, among them conversational UX for applications based on enterprise knowledge.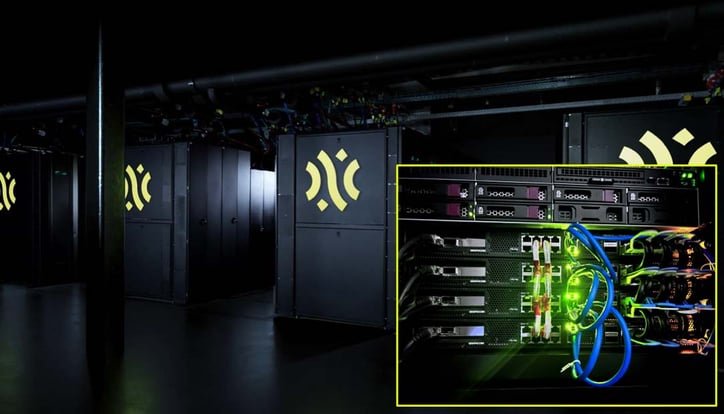
Graphcore IPUs in Aleph Alpha's alphaONE datacentre